7. 通用yolox模型部署¶
7.1. 引言¶
本文档介绍了如何将yolox架构的模型部署在cv181x开发板的操作流程,主要的操作步骤包括:
yolox模型pytorch版本转换为onnx模型
onnx模型转换为cvimodel格式
最后编写调用接口获取推理结果
7.2. pt模型转换为onnx¶
首先可以在github下载yolox的官方代码:[Megvii-BaseDetection/YOLOX: YOLOX is a high-performance anchor-free YOLO, exceeding yolov3~v5 with MegEngine, ONNX, TensorRT, ncnn, and OpenVINO supported. Documentation: https://yolox.readthedocs.io/ (github.com)](https://github.com/Megvii-BaseDetection/YOLOX/tree/main)
使用以下命令从源代码安装YOLOX
git clone git@github.com:Megvii-BaseDetection/YOLOX.git
cd YOLOX
pip3 install -v -e . # or python3 setup.py develop
需要切换到刚刚下载的YOLOX仓库路径,然后创建一个weights目录,将预训练好的.pth文件移动至此
cd YOLOX & mkdir weights
cp path/to/pth ./weigths/
【官方导出onnx】
切换到tools路径
cd tools
在onnx中解码的导出方式
python \
export_onnx.py \
--output-name ../weights/yolox_m_official.onnx \
-n yolox-m \
--no-onnxsim \
-c ../weights/yolox_m.pth \
--decode_in_inference
相关参数含义如下:
–output-name 表示导出onnx模型的路径和名称
-n 表示模型名,可以选择 * yolox-s, m, l, x * yolo-nano * yolox-tiny * yolov3
-c 表示预训练的.pth模型文件路径
–decode_in_inference 表示是否在onnx中解码
【TDL_SDK版本导出onnx】
为了保证量化的精度,需要将YOLOX解码的head分为三个不同的branch输出,而不是官方版本的合并输出
通过以下的脚本和命令导出三个不同branch的head:
将yolo_export/yolox_export.py复制到YOLOX/tools目录下,然后使用以下命令导出分支输出的onnx模型:
python \
yolox_export.py \
--output-name ../weights/yolox_s_9_branch_384_640.onnx \
-n yolox-s \
-c ../weights/yolox_s.pth
小技巧
如果输入为1080p的视频流,建议将模型输入尺寸改为384x640,可以减少冗余计算,提高推理速度,如下:
python \
yolox_export.py \
--output-name ../weights/yolox_s_9_branch_384_640.onnx \
-n yolox-s \
-c ../weights/yolox_s.pth \
--img-size 384 640
yolo_export中的脚本可以通过SFTP获取:下载站台:sftp://218.17.249.213 帐号:cvitek_mlir_2023 密码:7&2Wd%cu5k
通过SFTP找到下图对应的文件夹:
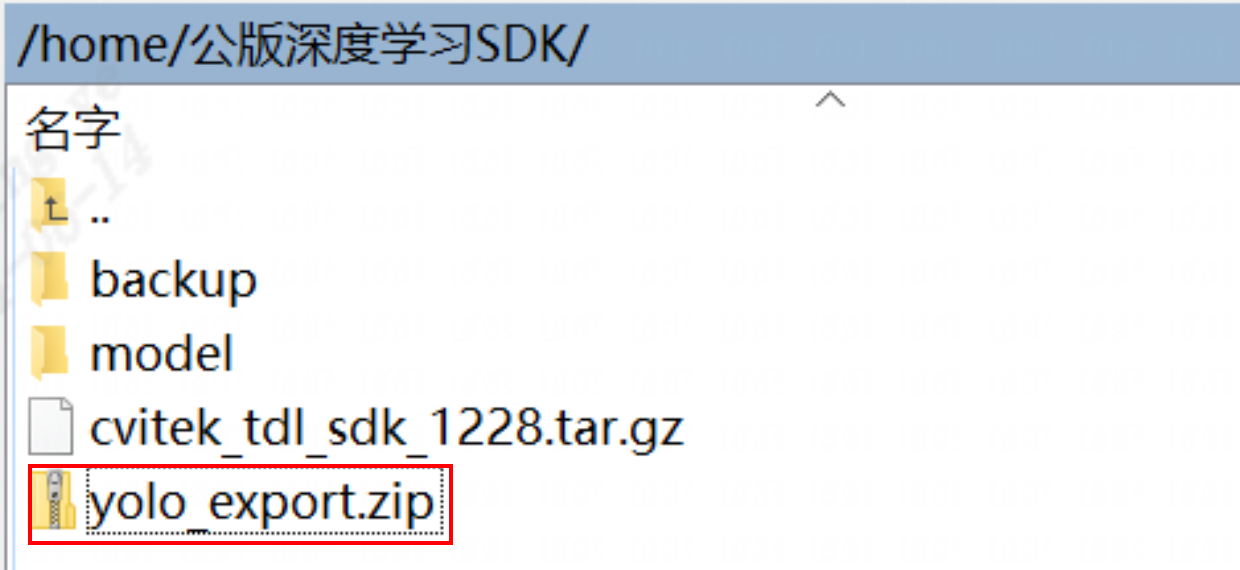
7.3. onnx模型转换cvimodel¶
cvimodel转换操作可以参考yolo-v5移植章节的onnx模型转换cvimodel部分。
7.4. TDL_SDK接口说明¶
### 预处理参数设置
预处理参数设置通过一个结构体传入设置参数
typedef struct {
float factor[3];
float mean[3];
meta_rescale_type_e rescale_type;
bool use_quantize_scale;
PIXEL_FORMAT_E format;
} YoloPreParam;
而对于YOLOX,需要传入以下四个参数:
factor 预处理scale参数
mean 预处理均值参数
use_quantize_scale 是否使用模型的尺寸,默认为true
format 图片格式,PIXEL_FORMAT_RGB_888_PLANAR
其中预处理factor以及mean的公式为 $$ y=(x-mean)times scale $$
### 算法参数设置
typedef struct {
uint32_t cls;
} YoloAlgParam;
需要传入分类的数量,例如
YoloAlgParam p_yolo_param;
p_yolo_param.cls = 80;
另外的模型置信度参数设置以及NMS阈值设置如下所示:
CVI_TDL_SetModelThreshold(tdl_handle, CVI_TDL_SUPPORTED_MODEL_YOLOX, conf_threshold);
CVI_TDL_SetModelNmsThreshold(tdl_handle, CVI_TDL_SUPPORTED_MODEL_YOLOX, nms_threshold);
其中conf_threshold为置信度阈值;nms_threshold为 nms 阈值
### 测试代码
#define _GNU_SOURCE
#include <stdio.h>
#include <stdlib.h>
#include <time.h>
#include <chrono>
#include <fstream>
#include <functional>
#include <iostream>
#include <map>
#include <sstream>
#include <string>
#include <vector>
#include "core.hpp"
#include "core/cvi_tdl_types_mem_internal.h"
#include "core/utils/vpss_helper.h"
#include "cvi_tdl.h"
#include "cvi_tdl_media.h"
#include "sys_utils.hpp"
int main(int argc, char* argv[]) {
int vpssgrp_width = 1920;
int vpssgrp_height = 1080;
CVI_S32 ret = MMF_INIT_HELPER2(vpssgrp_width, vpssgrp_height, PIXEL_FORMAT_RGB_888, 1,
vpssgrp_width, vpssgrp_height, PIXEL_FORMAT_RGB_888, 1);
if (ret != CVI_TDL_SUCCESS) {
printf("Init sys failed with %#x!\n", ret);
return ret;
}
cvitdl_handle_t tdl_handle = NULL;
ret = CVI_TDL_CreateHandle(&tdl_handle);
if (ret != CVI_SUCCESS) {
printf("Create tdl handle failed with %#x!\n", ret);
return ret;
}
printf("start yolox preprocess config \n");
// // setup preprocess
YoloPreParam p_preprocess_cfg;
for (int i = 0; i < 3; i++) {
p_preprocess_cfg.factor[i] = 1.0;
p_preprocess_cfg.mean[i] = 0.0;
}
p_preprocess_cfg.use_quantize_scale = true;
p_preprocess_cfg.format = PIXEL_FORMAT_RGB_888_PLANAR;
printf("start yolo algorithm config \n");
// setup yolo param
YoloAlgParam p_yolo_param;
p_yolo_param.cls = 80;
printf("setup yolox param \n");
ret = CVI_TDL_Set_YOLOX_Param(tdl_handle, &p_preprocess_cfg, &p_yolo_param);
printf("yolox set param success!\n");
if (ret != CVI_SUCCESS) {
printf("Can not set YoloX parameters %#x\n", ret);
return ret;
}
std::string model_path = argv[1];
std::string str_src_dir = argv[2];
float conf_threshold = 0.5;
float nms_threshold = 0.5;
if (argc > 3) {
conf_threshold = std::stof(argv[3]);
}
if (argc > 4) {
nms_threshold = std::stof(argv[4]);
}
printf("start open cvimodel...\n");
ret = CVI_TDL_OpenModel(tdl_handle, CVI_TDL_SUPPORTED_MODEL_YOLOX, model_path.c_str());
if (ret != CVI_SUCCESS) {
printf("open model failed %#x!\n", ret);
return ret;
}
printf("cvimodel open success!\n");
// set thershold
CVI_TDL_SetModelThreshold(tdl_handle, CVI_TDL_SUPPORTED_MODEL_YOLOX, conf_threshold);
CVI_TDL_SetModelNmsThreshold(tdl_handle, CVI_TDL_SUPPORTED_MODEL_YOLOX, nms_threshold);
std::cout << "model opened:" << model_path << std::endl;
VIDEO_FRAME_INFO_S fdFrame;
ret = CVI_TDL_ReadImage(str_src_dir.c_str(), &fdFrame, PIXEL_FORMAT_RGB_888);
std::cout << "CVI_TDL_ReadImage done!\n";
if (ret != CVI_SUCCESS) {
std::cout << "Convert out video frame failed with :" << ret << ".file:" << str_src_dir
<< std::endl;
}
cvtdl_object_t obj_meta = {0};
CVI_TDL_YoloX(tdl_handle, &fdFrame, &obj_meta);
printf("detect number: %d\n", obj_meta.size);
for (uint32_t i = 0; i < obj_meta.size; i++) {
printf("detect res: %f %f %f %f %f %d\n", obj_meta.info[i].bbox.x1, obj_meta.info[i].bbox.y1,
obj_meta.info[i].bbox.x2, obj_meta.info[i].bbox.y2, obj_meta.info[i].bbox.score,
obj_meta.info[i].classes);
}
CVI_VPSS_ReleaseChnFrame(0, 0, &fdFrame);
CVI_TDL_Free(&obj_meta);
CVI_TDL_DestroyHandle(tdl_handle);
return ret;
}
7.5. 测试结果¶
测试了yolox模型onnx以及在cv181x/2x/3x各个平台的性能指标,其中参数设置:
conf: 0.001
nms: 0.65
分辨率:640 x 640
yolox-s模型的官方导出方式性能:
测试平台 |
推理耗时 (ms) |
带宽 (MB) |
ION(MB) |
MAP 0.5 |
MAP 0.5-0.95 |
---|---|---|---|---|---|
pytorch |
N/A |
N/A |
N/A |
59.3 |
40.5 |
cv180x |
ion分配失败 |
ion分配失败 |
37.41 |
量化失败 |
量化失败 |
cv181x |
131.95 |
104.46 |
16.43 |
量化失败 |
量化失败 |
cv182x |
95.75 |
104.85 |
16.41 |
量化失败 |
量化失败 |
cv183x |
量化失败 |
量化失败 |
量化失败 |
量化失败 |
量化失败 |
cv186x |
12.39 |
89.47 |
19.56 |
量化失败 |
量化失败 |
yolox-s模型的TDL_SDK导出方式性能:
测试平台 |
推理耗时 (ms) |
带宽 (MB) |
ION(MB) |
MAP 0.5 |
MAP 0.5-0.95 |
---|---|---|---|---|---|
onnx |
N/A |
N/A |
N/A |
53.1767 |
36.4747 |
cv180x |
ion分配失败 |
ion分配失败 |
35.21 |
ion分配失败 |
ion分配失败 |
cv181x |
127.91 |
95.44 |
16.24 |
52.4016 |
35.4241 |
cv182x |
91.67 |
95.83 |
16.22 |
52.4016 |
35.4241 |
cv183x |
30.6 |
65.25 |
14.93 |
52.4016 |
35.4241 |
cv186x |
11.39 |
63.17 |
19.48 |
52.61 |
35.49 |
yolox-m模型的官方导出方式性能:
测试平台 |
推理耗时 (ms) |
带宽 (MB) |
ION(MB) |
MAP 0.5 |
MAP 0.5-0.95 |
---|---|---|---|---|---|
pytorch |
N/A |
N/A |
N/A |
65.6 |
46.9 |
cv180x |
ion分配失败 |
ion分配失败 |
92.41 |
ion分配失败 |
ion分配失败 |
cv181x |
ion分配失败 |
ion分配失败 |
39.18 |
量化失败 |
量化失败 |
cv182x |
246.1 |
306.31 |
39.16 |
量化失败 |
量化失败 |
cv183x |
量化失败 |
量化失败 |
量化失败 |
量化失败 |
量化失败 |
cv186x |
30.55 |
178.98 |
38.72 |
量化失败 |
量化失败 |
yolox-m模型的TDL_SDK导出方式性能:
测试平台 |
推理耗时 (ms) |
带宽 (MB) |
ION(MB) |
MAP 0.5 |
MAP 0.5-0.95 |
---|---|---|---|---|---|
onnx |
N/A |
N/A |
N/A |
59.9411 |
43.0057 |
cv180x |
ion分配失败 |
ion分配失败 |
92.28 |
ion分配失败 |
ion分配失败 |
cv181x |
ion分配失败 |
ion分配失败 |
38.95 |
N/A |
N/A |
cv182x |
297.5 |
242.65 |
38.93 |
59.3559 |
42.1688 |
cv183x |
75.8 |
144.97 |
33.5 |
59.3559 |
42.1688 |
cv186x |
33.05 |
173.20 |
38.64 |
59.34 |
42.05 |